Learning from Nature
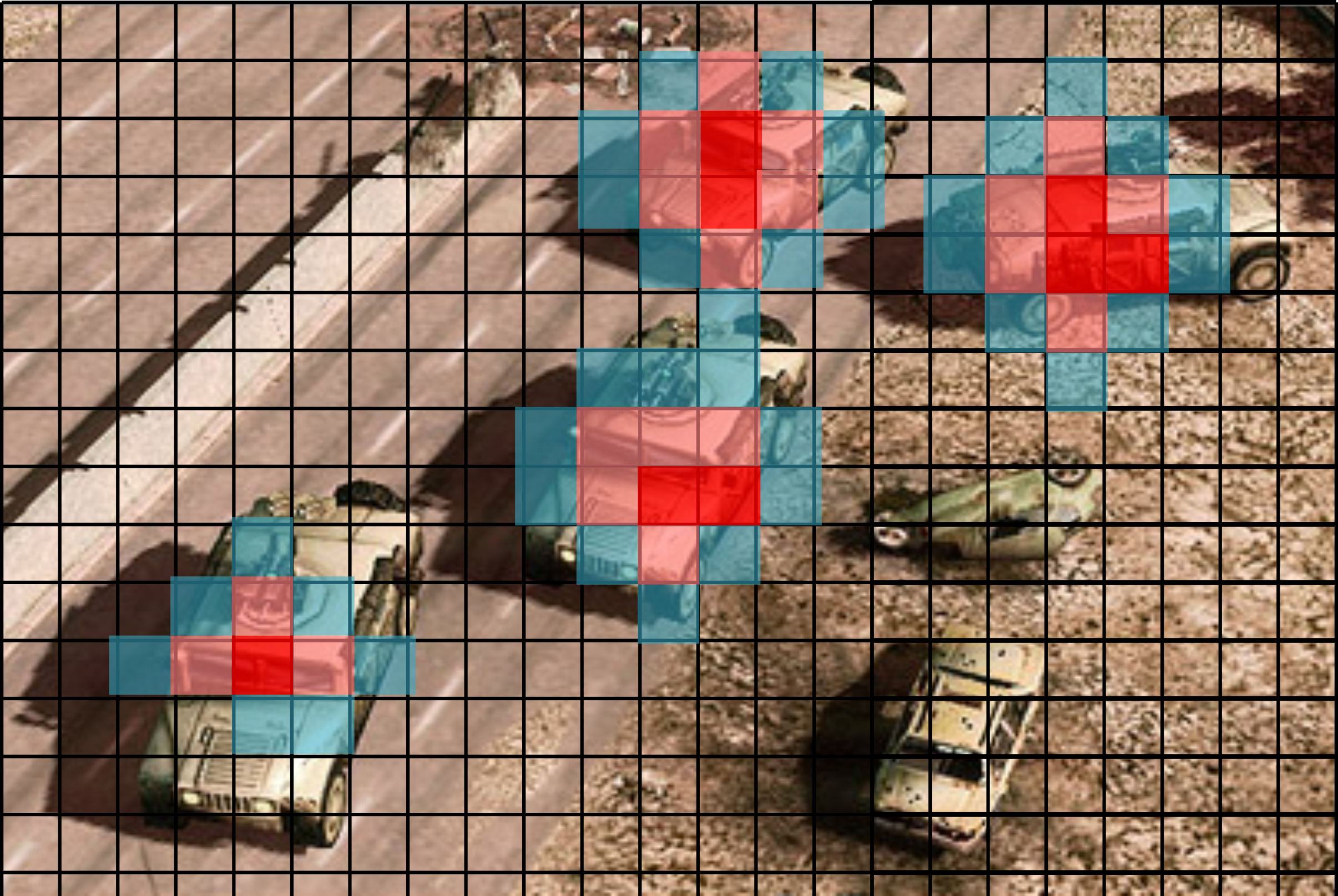
Porpoises do it. Bats do it. Why can’t autonomous vehicles do it? No, not fall in love, but navigate with sonar. A research team is developing the foundational technology that mimics how animals use sonar, called biosonar, to improve navigation for robots.
Traditional navigation tools, like Light Detection and Ranging (LIDAR) and cameras, falter in stormy weather conditions and have a limited range of application. Sonar (sound navigation and ranging) has offered the sciences a stealthy, low-cost and energy-efficient alternative for navigation for decades, but this technology has limitations.
When a sound wave encounters a target, the waves reflect from the object’s surface forming a series of echoes. These reflecting waves move in different directions and at different angles. As one moves farther from the object, the echoes overlap and merge, producing a cacophony of high-frequency waves to deconstruct and interpret. The research team has turned to nature, in particular to dolphins, to understand how animals have solved this problem.
“We want to use the same approach as dolphins,” said Bogdan Popa, assistant professor of Mechanical Engineering at the University of Michigan and lead investigator on the project. “Dolphins are so much better than engineered systems at interpreting the echoes.”
“Synthetic environments are becoming the most popular approach for the development of complex algorithms for autonomous vehicles, including new sensors for perception,” said Bogdan Epureanu, Arthur F. Thurnau professor of Mechanical Engineering and co-investigator on the project. “To understand how to use an approach such as those used by dolphins, surrogate measurement data is needed to pre-train machine learning algorithms.” Therefore, the team will explore biosonar-based perception algorithms in synthetic environments such as those used in the gaming industry.
To begin, the team will create a model to simulate how high-frequency sound waves propagate through a synthetic environment. The model will provide a way to describe the geometric path the waves follow after they reflect off the surface of a target. Using these geometries, the team hopes to classify objects based on a unique echo map. The team will then use a probe at different distances to test the ‘known’ target map for accuracy.
“There is a school of thought in neuroscience that believes the biological brain follows the same processes as convolutional neural networks,” said Popa. “We are excited to test this hypothesis and want to train convolutional neural networks to recognize certain objects, like cars, from the echoes of pulses that bounce off objects.”
A convolutional neural network (CNN) is a deep learning algorithm that can differentiate, weigh, and assign importance to images using self-labeled data obtained from the first stage of the study in the synthetic environment. Popa hopes to develop a CNN that replicates biosonar by storing target information in the navigational memory consisting of echo maps obtained during training sessions.
Popa and his team will evaluate how the probe works in a cluttered real-world environment to disentangles the overlapping echoes produced by known targets and random objects. Here, the team will turn to animals to figure out how nature has solved this problem. For example, marine mammals use the side of the ultrasound beam to discriminate between objects in a cluttered environment and constantly compare received echoes to the memory maps for target objects.
The results from this study could also offer opportunities for civilian benefit. According to Popa, any advances from this study could be applied to other imaging technologies, such as medical ultrasound machines.
###
Popa was joined by Bogdan Epureanu and Hyung-Suk Kwon at UMich, Paramsothy Jayakumar at the Ground Vehicle System Center and Paul Mohan at ZF Group on the project, titled “Perception in complex scenes using automatically labeled sonar-imaging data in synthetic environments.” The project received funding from Ground Vehicle System Center through the Automotive Research Center at the University of Michigan.
-–
Stacey W. Kish