Helping Autonomous Vehicles Learn
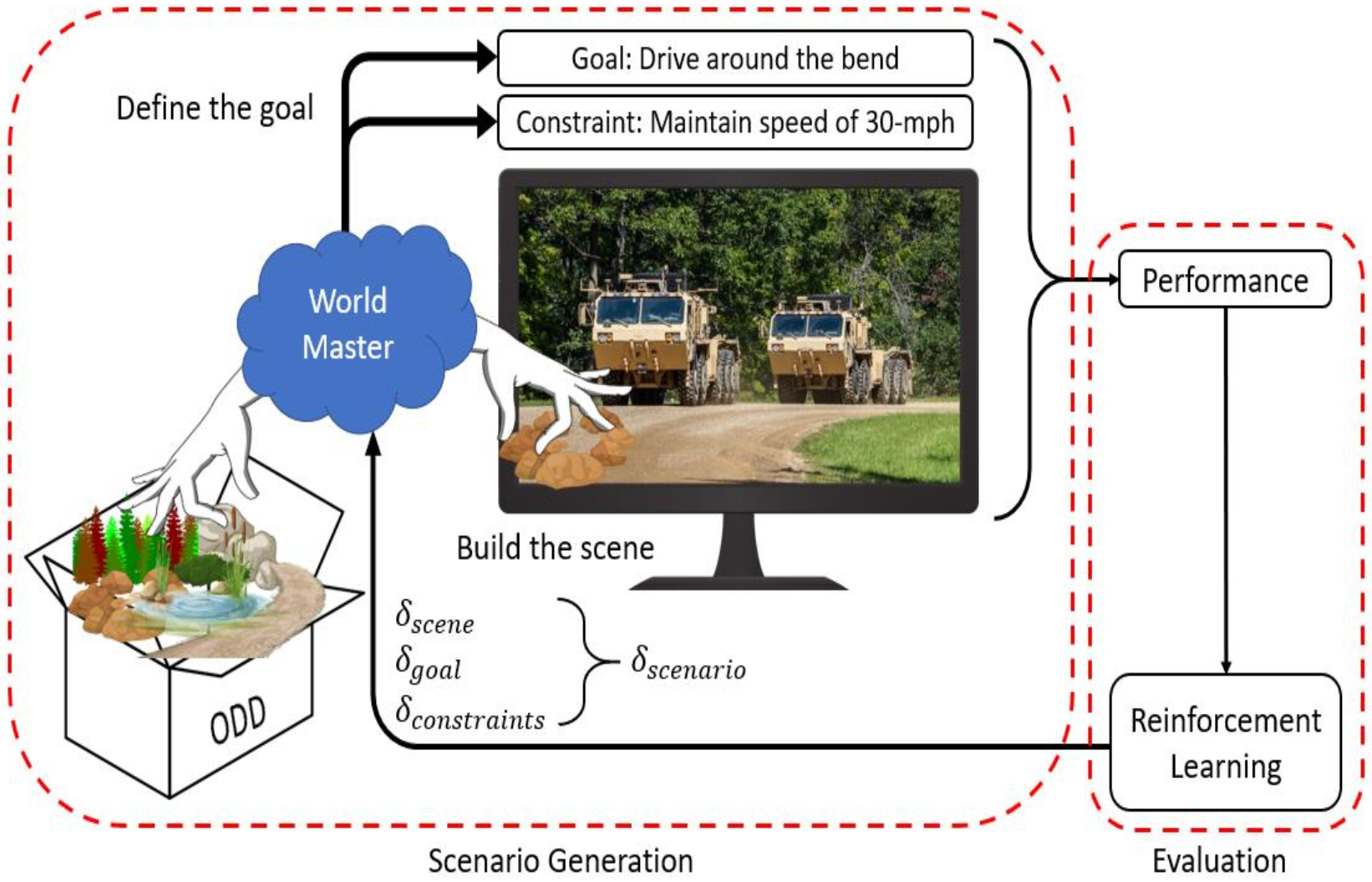
“If you want to make a good algorithm for playing chess even better, one way to do it is to let the algorithm play against itself,” said Ram Vasudevan, assistant professor of Mechanical Engineering at UMich. “By playing against itself, the algorithm can learn how to maneuver more successfully through the game.”
Autonomous vehicles use algorithms to ‘see’ the surrounding environment. Methods to evaluate the performance of these algorithms in their task remains lacking. A group of scientists at the University of Michigan are turning to the ultimate game of strategy to help algorithms ‘learn’ from past mistakes.
Vasudevan and his team are developing simulated environments to train autonomous military vehicles to evaluate terrain more effectively to navigate through an unstructured environment with greater success.
In the absence of roads or worn paths, autonomous vehicles must make crucial decisions on the best path to travel to get from point A to point B. As a result, these vehicles constantly evaluate the surrounding landscape to decide whether or not to drive through, say muddy terrain, or around it.
At the moment, scientists are unsure of how to verify that these algorithms will behave correctly in arbitrary environments. Vasudevan and his team are working to develop realistic simulations to test algorithms and help them ‘see’ the surrounding environment with greater accuracy when navigating through an uncharted region.
“Autonomous vehicles have to traverse a landscape that may not be well manicured,” said Vasudevan. “We are creating a simulated world that can fool an autonomous system to see whether it makes bad decisions.”
The team is translating how humans see the world into a series of metrics to create the simulated world. Their work is creating a variety of environments from mud to ice and snow, to a landscaped littered with debris. The simulated environment consists of maps of images that the autonomous vehicle can evaluate as it navigates through this world. If autonomous system can detect that it is being fooled, then it has learned to navigate the complicated scenarios successfully.
Vasudevan believes that any advances in this project can be applied to the civilian setting. The lessons learned from training autonomous vehicles to navigate through natural territory can be applied to autonomous vehicles that move through a structured environment that has roads, bridges, and rules of the road.
###
Vasudevan is joined on this project by Bogdan Epureanu at UMich, Mike Del Rose, Denise Rizzo, and Matt Castanier at the U.S. Army Ground Vehicle Systems Center, Seunghun Baek at Ford Motor Company, and Ted Sender, a student at UMich. The project “Verification of Deep Learning Algorithms for Autonomy Related Tasks” received funding through the Automotive Research Center.
Stacy W. Kish