Discovering New Materials for Thermal Energy Storage
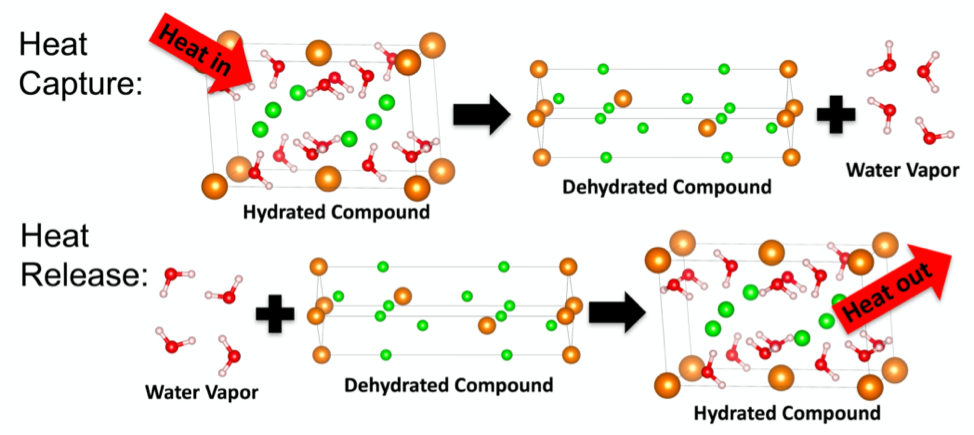
Of the energy consumed annually in the United States, roughly two-thirds does no useful work and is ultimately lost in the form of heat. In passenger vehicles, tire and engine friction and wind resistance, as examples, contribute to these inefficiencies.
Devising ways to remove heat can also be desirable. For example, in military ground vehicles, high-traction efforts like driving through sand generate excess heat that can overheat transmission fluid and lead to transmission failure.
But what if the thermal energy from these applications could be captured, stored, and reused? Don Siegel, professor of mechanical engineering and an ARC principal investigator, is using high-throughput computational methods to do just that — identifying promising materials for the capture, storage and release of thermal energy.
“Improved thermal energy storage could make many processes and devices more efficient,” Siegel said.
Potential applications include solar energy storage, temperature control of electronic devices and waste heat capture from vehicles as well as wearables to help soldiers stay warm in cold conditions or cool in hot conditions.
Looking to thermochemical storage
Siegel’s group focuses on thermochemical storage, a particular type of heat storage that relies on chemical reactions, rather than on simple temperature or phase changes. It has the potential to achieve very high energy densities, and the reactions associated with the storage process can be reversible. More specifically, Siegel’s group is looking at hydration reactions, which involve water absorbing and desorbing from a solid, such as a salt.
In this approach, energy is stored by supplying heat to the hydrate material. This heat causes the water contained within the solid to evaporate. Energy can then be stored indefinitely by keeping the water and dehydrated salt separate. Mixing the two reverses the process and releases heat.
Unfortunately, optimizing this process is a challenge, because too many potential hydrates exist to analyze them all using conventional experiments. So Siegel’s team is using high-throughput computation and machine learning to discover optimal hydrates and to characterize their reactions.
Mining known hydrates
Using the Inorganic Crystal Structure Database and U-M’s Great Lakes high-performance computing cluster, Siegel’s group performed quantum mechanical calculations to screen hundreds of experimentally-known hydrates for their stability and energy storage capacity.
The team’s early work characterized almost 300 hydration reactions, some 150 of which had not been previously reported. These computational results were validated with experimental data from previous investigations published in the literature.
The findings led Siegel to a new hypothesis: There could be many more promising hydrates that have not yet been synthesized.
To explore this possibility, the team generated over 5,000 “hypothetical” hydrates (and their dehydrated salts) and almost 3,800 reactions. This was done using the known salt hydrate crystal structures from their earlier work as templates, and then populating these templates with different atoms through appropriate substitutions. The stability and energy density of each material was subsequently computed. Of these, the team identified several additional promising reactions with high energy densities and narrow operating temperature windows.
To put the performance of these new materials in context, the team compared their capacities to that of a lithium-ion (Li-ion) battery. To facilitate an apples-to-apples comparison, the battery, which stores energy as electricity rather than heat, was stripped of all components except its active materials.
The results were encouraging.
“Accounting for the conversion of heat to electricity, the best-performing TES materials are smaller and lighter than batteries at low temperatures,” said Siegel, who has filed invention disclosures on some of the new materials.
Leveraging machine learning
The team is again extending their search, this time with the help of machine learning.
By combining the data from their prior studies, Siegel’s group had created a database of thousands of hydrates that includes reaction temperatures, crystal structures and other properties. Current work is focused on training machine learning (ML) algorithms to identify the most important features of, and making rapid predictions about, the TES potential of new hydrates — without the need for time-intensive quantum-mechanical calculations.
To date, the group has focused on salt hydrates that contain only a single metal ion. But using the crystal structures from both single- and mixed-metal hydrates and performing similar isoelectronic substitutions yields nearly 800,000 mixed-metal hydrate candidates.
“To make predictions at this scale requires that we go beyond brute-force screening — high throughput calculations aren’t fast enough. Fortunately, it appears that that machine learning will help us bridge the gap,” Siegel said.
The team has shown some promising results using ML to make rapid predictions based on a few elementary inputs. The group’s ultimate aim is to identify design rules for new stable, high-capacity TES materials.
Future work will also extend the screening approach to metal-organic frameworks (MOFs), porous materials with extremely high surface areas that make them useful for the types of hydration reactions the team is pursuing, as well as to predicting reaction rates. These rate predictions are less amenable to high-throughput analysis.
As with salt hydrates, the team has a large database of MOFs ready to explore. Building upon the materials discovery work, next steps also include analyzing the benefits to prototype TES systems that have been built around the enhanced storage media the team has discovered.
The research team includes Steven Kiyabu, a doctoral candidate in Mechanical Engineering (ME); Alauddin Ahmed, research scientist in Siegel’s Energy Storage and Materials Simulation Lab; Daewoong Kim, a master’s student in ME; and ME undergraduate Patrick Girard.
As the first to demonstrate a computational screening protocol for TES materials, Siegel sees tremendous opportunity. “Computation is often used to help us understand, but it’s even more powerful when it can make predictions. In the context of TES, these predictions are leading us to new and better materials.”
Publications:
Computational Screening of Hydration Reactions for Thermal Energy Storage: New Materials and Design Rules. Chemistry of Materials. 2018, 30, 2006–2017.
Stacy W. Kish