Data Is Power
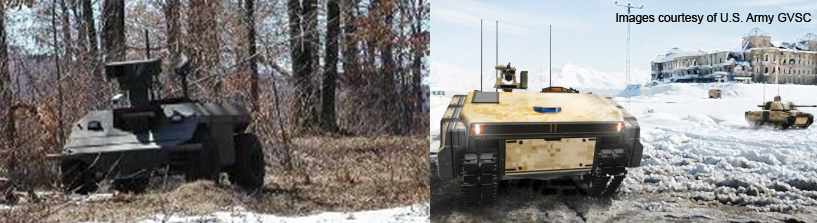
Army vehicles often operate in unstructured surroundings, like a battlefield, under many different environmental conditions. When both manned and autonomous vehicles go into the field, information is gathered. Along with GPS coordinates, gear changes, user interventions, RPMs and tractive effort, video provides reams of data to describe the environment and conditions that ultimately led to the operation’s failure. Machine learning is the mechanism that robotic systems use to screen information obtained in the field to improve future performance. At this time, this approach requires data to be labeled manually, which is both laborious and expensive.
“In a battlefield environment, vehicles have to recognize friendly allies compared to obstacles or hostile forces that will impair the operation,” said Nickolas Vlahopoulos, professor of Naval Architecture and Marine Engineering at the University of Michigan. “We would like to create capability to recognize certain items in the field, like certain types of assets, obstacles, etc. (relevant classes), and take corrective action to avoid disruption during an operation.”
Vlahopoulos and his research team are circumventing normal labeling procedures by fast-tracking information processing to improve vehicle performance during future operations. The team is developing a new method that has the potential to identify relevant objects in the images on each frame of the video collected in the field. It will also be able to determine when an encountered object does not belong to one of the relevant classes (irrelevant object).
Their research developments are focusing in two areas. The feature extractor is trained in an unsupervised manner using unlabeled data collected from the operational environment. Once the weights of the filters of the feature extractor are available, only a limited number of labeled images for the relevant objects and unlabeled images for the irrelevant objects will be used for training a classifier. The latter will be capable of recognizing relevant classes, like vehicles, military personnel, and other military equipment that might be encountered in the field, and it will also know when irrelevant objects are present.
The research for developing the new classifier has been completed and tested. It offers a unique solution to generating low-label and low-shot learning capabilities. This approach provides the classifier training without spending a lot of time labeling. He found this method improved identification accuracy by up to 30% for images not grouped into any specific classes, as well as retaining the ability to identify items of interest. With the ‘knowledge’ gained with this method, engineers can develop algorithms to produce strategies for vehicles to navigate similar operational conditions in the field successfully.
Currently the research is continuing on training the feature extractor in an unsupervised manner while using images from typical Army operational environments.
###
Vlahopoulos was joined by Spyros Kasapis at UMich, William Tecos at General Dynamics, Hongyoon Kim at Samsung Electronics, and Ryan Kreiter, Patel Sahill, and Paramsothy Jayakumar with the U.S. Army Combat Capabilities Development Command Ground Vehicle Systems Center on the project titled, “Processing image data from unstructured environments.” This project received funding from the Automotive Research Center at UMich in accordance with the Cooperative Agreement with the U.S. Army CCDC Ground Vehicle Systems Center.
Stacy W. Kish