Systems of Systems & Integration
Annual PlanOptimal Crowdsourcing Framework for Engineering Design
Project Team
Government
Richard Gerth, Andrew Dunn, Lisa Graf, Pradeep Mendonza, U.S. Army GVSC
Faculty
Honglak Lee, Rich Gonzalez, Max Yi Ren, University of Michigan
Industry
Damien DeClercq, Local Motors
Student
Alex Burnap, University of Michigan
Project Summary
Work began in 2012 and reached completion in 2015.
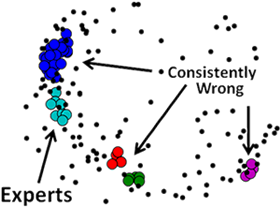
Crowdsourcing is a widely publicized (and somewhat misunderstood) method of utilizing a group of individuals to perform tasks. The task can range from evaluating items in consumer reviews to design and manufacturing of a complex system, e.g., the DARPA AVM. Studies on crowdsourcing practices have been reported to show the advantage of crowdsourcing against conventional problem solving, including design, due to the diversity of expertise within the crowd.
Most crowdsourcing efforts to date are performed in an ad hoc manner. In this project, we proposed to initiate a rigorous study of crowdsourcing as a design process by combining research knowledge from systems design engineering, computer science and behavioral science. An early question in such a research endeavor is whether crowdsourcing can be reliable in supporting serious decision making – and under what conditions. Focusing more deeply, we want to study what could be the properties of the crowd (such as composition and size) to achieve such reliability. To this end, the first year of this project focused on creating a simulation environment for the crowd and the crowdsourcing process in order to explore how properties of the design problem and properties of the crowd affect the crowd’s ability to perform good evaluation tasks – before collecting actual human data.
A crowd can help evaluate and validate a design based not only using given simulation results for physics-based performance criteria, but using also criteria that cannot be captured by simulations, often related to subjective but important human judgments. Linking physics-based simulation evaluations with human-based evaluations in a formal rigorous manner greatly expands our design capabilities. Note that a crowd here may be a collection of very diverse individuals, from design engineers, to generals and soldiers to maintenance workers.
The research investigated how well a crowd can evaluate design performance criteria. The evaluation task was selected because it is amenable to mathematical modeling, and evaluation is an important part of a crowd sourcing design process. More specific questions were: (1) Under what circumstances is crowdsourcing be more effective than a conventional design team; (2) for a given design task, what are preferred ways to present the task to the crowd; and (3) what are appropriate crowd structures (crowd composition and individual properties) in terms of diversity, expertise, and crowd member roles.
Publications:
R. Gerth, A. Burnap, P. Y. Papalambros, “Crowdsourcing: A Primer and its Implications for Systems Engineering”, Proceedings of the NDIA Ground Vehicle Systems Engineering and Technology Symposium (GVSETS), Troy, MI, Aug 14-Aug 16, 2012.
Y. Ren, P. Y. Papalambros, “On Design Preference Elicitation with Crowd Implicit Feedback”, ASME International Design Engineering Technical Conferences, DETC2012-70605, Chicago, Aug 12-Aug 15, 2012.
Burnap, Alex, Ren, Yi, Papalambros, Panos Y., Gonzalez, Richard, and Gerth, Richard. “A Simulation Based Estimation of Crowd Ability and its Influence on Crowdsourced Evaluation of Design Concepts.” Proceedings of the ASME 2013 International Design Engineering Technical Conferences and Computers and Information in Engineering Conference. Volume 3B: 39th Design Automation Conference. Portland, Oregon, USA. August 4–7, 2013. V03BT03A004. ASME.
Ren, Yi, Scott, Clayton, and Papalambros, Panos Y. “A Scalable Preference Elicitation Algorithm Using Group Generalized Binary Search.” Proceedings of the ASME 2013 International Design Engineering Technical Conferences and Computers and Information in Engineering Conference. Volume 3B: 39th Design Automation Conference. Portland, Oregon, USA. August 4–7, 2013. V03BT03A005. ASME.
Ren, Y., Burnap, A., & Papalambros, P. (2013). Quantification of perceptual design attributes using a crowd. In Proceedings of the 19th International Conference on Engineering Design: Design for Harmonies, ICED 2013 (Vol. 6 DS75-06, pp. 139-148)
Burnap, A., Gerth, R., Gonzalez, R., and Papalambros, P. Y., “Identifying Experts in the Crowd for Evaluation of Engineering Designs”, Journal of Engineering Design, 28:5, 317-337, 2017.
Burnap, Alex, Ren, Yi, Lee, Honglak, Gonzalez, Richard, and Papalambros, Panos Y. “Improving Preference Prediction Accuracy With Feature Learning.” Proceedings of the ASME 2014 International Design Engineering Technical Conferences and Computers and Information in Engineering Conference. Volume 2A: 40th Design Automation Conference. Buffalo, New York, USA. August 17–20, 2014. V02AT03A012. ASME.
Burnap, A., Ren, Y., Gerth, R., Papazoglou G., Gonzalez, R., and Papalambros, P. Y., “When Crowdsourcing Fails: A Study of Expertise on Crowdsourced Design Evaluation,” Journal of Mechanical Design, 137.3, 2015.