Systems of Systems & Integration
Annual PlanModeling and Simulation of Repairable Systems for Depot Maintenance and Warranty Forecasting using an Effective Age Approach
Project Team
Principal Investigator
Zissimos Mourelatos, Oakland University Vijitashwa Pandey, Oakland UniversityGovernment
Matthew Castanier, Amandeep Singh, Monica Majcher, U.S. Army GVSC
Clifton Ellis, PEO CS&CSS
Industry
Adamantios Mettas, HBM Prenscia (nCode & ReliaSoft)
Mohammad Hijawi, FCA
Student
Themistoklis Koutsellis, Oakland University
Project Summary
Work spanned two projects from 2012-2015 and 2016-2018.
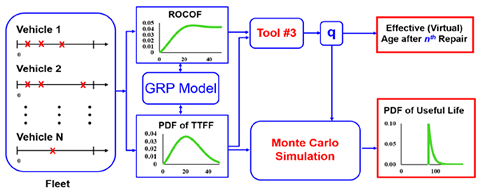
Most engineering systems are repairable. Their components can be repaired or renewed, if system failure occurs, so that the system can be put back into service. The classical reliability theory however, captures only limited aspects of the performance of these systems. Reliability may become equal to one after repairs. Based on the timing and quality of repairs, different reliability curves can be realized for the same system. The commonly used metrics of Mean-Time-Between-Failures (MTBF) and availability do not provide complete statistical information. MTBF is a widely used concept but only captures the mean. Availability may be misleading because a system that needs constant repair but takes only a short time to repair has a very high availability. Such a system however, has a very small practical use. These observations indicate that classical reliability theory may be inadequate in representing the performance of repairable systems. Over time, many components in a system get old or repaired and the frequency of system breakdown increases. Therefore, a predefined planning horizon (or lifecycle) must be considered. Repairs are performed only within the planning horizon and the system is retired at the end of the planning horizon.
In this project, we developed techniques to deduce the system architecture (reliability block diagram) from limited data, and also determine 1) which system architectures lead to the best cost-performance tradeoff, 2) what performance metrics can best assess the reliability of a repairable system, and 3) how we can manage spare-parts inventory so that a repairable system can be put back to use with minimal interruption without carrying too much inventory and without frequent resupply. We explored a utility-theoretic method and a Pareto front method for the optimal design of a repairable system. Repair assumptions such as “same-as-old,” “good-as-new,” “better-than-old-but-worse-than-new” and “worse-than-old” as well as technical obsolescence are accounted for using the concept of effective age. These repair assumptions are essential for depot maintenance and optimal operation of repairable systems.
Modeling technical obsolescence and repair assumptions is directly relevant to the implementation of “reset.” The topic is of high interest to the Army in general, and to PEO CS & CSS (Combat Support and Combat Service Support) in particular, because most ground vehicle fleets will continue to be used for decades and the Army needs tools to help make fleet planning decisions and develop depot maintenance strategies that will help reduce O&S costs while ensuring that the vehicle fleets maintain their required performance levels.
All developments are incorporated into TARDEC’s Fleet Maintenance Simulation (FMS) software tool, which simulates the performance and maintenance actions required for a vehicle fleet in order to predict reliability, availability, and maintainability, as well as to carry out subsequent tradeoff studies, sensitivity analyses, and design optimization.
Publications:
- T. Koutsellis, Z.P. Mourelatos, M. Hijawi, H. Guo and M. Castanier, “Warranty Forecasting of Repairable Systems for Different Production Patterns,” SAE International Journal of Materials & Manufacturing, 10(3), 2017. Also in Proceedings of SAE World Congress, Detroit, MI, Paper# 2017-01-0209, 2017.
- T. Koutsellis, Z.P. Mourelatos and M. Majcher, Numerical Estimation of Expected Number of Failures for Repairable Systems Using a Generalized Renewal Process Model.” ASME J. Risk Uncertainty Part B. June 2019; 5(2): 020904, April 2019.
- T. Koutsellis and Z.P. Mourelatos, “Parameter Estimation of Limited Failure Population Model with a Weibull Underlying Distribution,” ASME J. Risk Uncertainty Part B., Sep 2019 (Accepted).