Intelligent Power Systems
Annual PlanComputational Discovery of Materials for Energy Storage: High Throughput Screening and Machine Learning
Project Team
Government
Denise Rizzo, In-Ho Lee, U.S. Army GVSC
Faculty
Alauddin Ahmed, University of Michigan
Industry
Mike Veenstra, Ford Motor Company
Student
Steven Kiyabu, University of Michigan
Project Summary
Ongoing project that started in 2018.
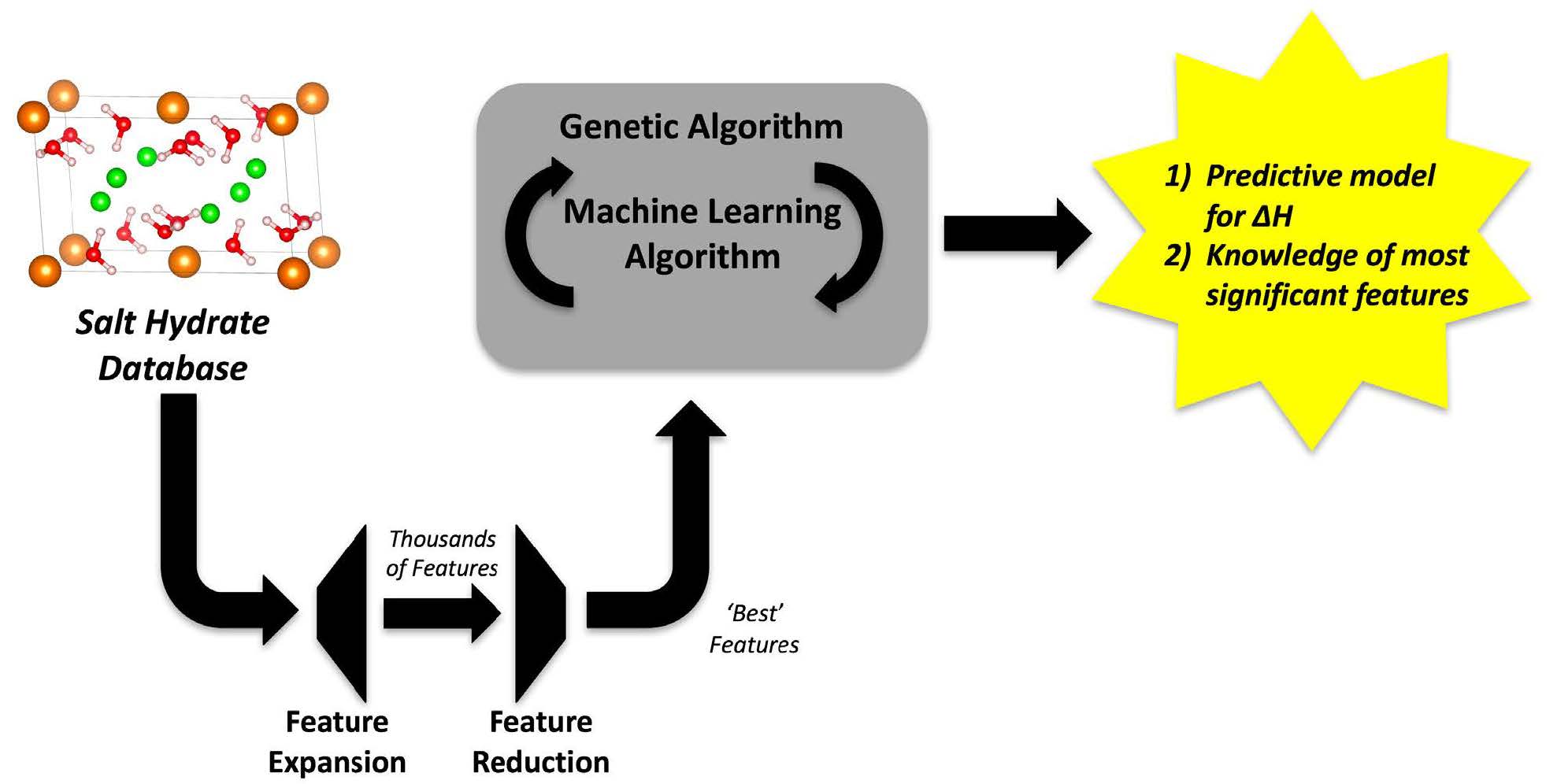
This project aims to discover and design efficient materials for thermal energy storage (TES) using data-centric computational methods. Emphasis will be given to storage via thermochemical means – employing reversible water uptake/release reactions – in hydrates, hydroxides, and metal-organic frameworks. These materials have the potential to achieve thermal energy densities that surpass other materials classes, yet have not been systematically explored using predictive computational techniques. An existing high-throughput computational workflow will be extended to screen thousands of real and hypothetical compounds with respect to their thermal capacities and thermodynamic properties. The resulting synthetic database linking properties and performance will be analyzed using machine learning techniques. These analyses will reveal design rules compatible with high TES performance, and allow for the development of models that can rapidly predict performance using only a small number of elementary materials properties as input.
The identification of new materials for thermal energy storage is well-described by the venerable ‘needle in a haystack’ analogy: many more compounds exist than can be practically investigated using conventional experimental or computational techniques. This project will circumvent this bottleneck by employing a combination of high-throughput computational prediction and machine learning analyses. One meritorious outcome of this approach will be the identification best-in-class TES materials. A second outcome is enhanced understanding: at present, the fundamental materials properties that make for a ‘good’ (or poor) TES material are unknown. By establishing guidelines for the design of these materials, a rational approach to their development can be pursued.
Publications:
- S. Kiyabu, J. S. Lowe, A. Ahmed, and D. J. Siegel, Computational Screening of Hydration Reactions for Thermal Energy Storage: New Materials and Design Rules, Chemistry of Materials, 30, 2006-2017 (2018).
doi: 10.1021/acs.chemmater.7b05230
Recognized with both an ACS Editors’ Choice Award and an ACS ‘Most Read’ article designation - Kiyabu, S.; Ahmed, A.; Siegel, D. J. (2018). High-Capacity Thermal Energy Storage Materials: Computational Screening and Machine Learning Analysis. Presentation at the 2018 Materials Research Society Fall Conference, Boston, MA.